
Corporate Credit Risk Modeling Training Course
Edstellar's instructor-led Credit Risk Modeling training course equips teams with analytical and predictive modeling skills to optimize financial decision-making and enhance organization compliance. The course enables employees to manage lending risks and set appropriate credit limits, enhancing their organizations' financial stability.
(Virtual / On-site / Off-site)
Available Languages
English, Español, 普通话, Deutsch, العربية, Português, हिंदी, Français, 日本語 and Italiano
Drive Team Excellence with Credit Risk Modeling Corporate Training
Empower your teams with expert-led on-site/in-house or virtual/online Credit Risk Modeling Training through Edstellar, a premier Credit Risk Modeling training company for organizations globally. Our customized training program equips your employees with the skills, knowledge, and cutting-edge tools needed for success. Designed to meet your specific training needs, this Credit Risk Modeling group training program ensures your team is primed to drive your business goals. Transform your workforce into a beacon of productivity and efficiency.
Credit Risk Modeling is the statistical analysis and prediction of the likelihood of a borrower defaulting on a loan or credit obligation, which is crucial for financial institutions to assess and manage their exposure to potential losses. Organizations rely on Credit Risk Modeling to make informed decisions about lending, investment, and risk management, helping them optimize profitability while minimizing the likelihood of default and financial losses. Credit Risk Modeling training course typically involves mastering statistical techniques, financial modeling, and data analysis to assess and mitigate credit risks in various lending scenarios effectively.
Edstellar's instructor-led Credit Risk Modeling training course is designed to cater to global teams through virtual/onsite training sessions. Delivered by industry experts with extensive experience, the training offers a customized curriculum that blends practical knowledge with theoretical frameworks.

Key Skills Employees Gain from instructor-led Credit Risk Modeling Training
Credit Risk Modeling skills corporate training will enable teams to effectively apply their learnings at work.
- Statistical AnalysisStatistical Analysis involves collecting, interpreting, and presenting data to identify trends and patterns. This skill is important for data-driven roles, enabling informed decision-making and strategic planning.
- Probability TheoryProbability Theory is the mathematical study of uncertainty, enabling professionals to analyze data, make predictions, and inform decision-making. this skill is important for roles in data science, finance, and risk management, as it helps in assessing risks and optimizing outcomes.
- Data ModelingData Modeling is the process of creating visual representations of data structures and relationships. This skill is important for data analysts and database developers to ensure accurate data management and analysis.
- Portfolio ManagementPortfolio Management is the art of strategically managing a collection of investments to achieve specific financial goals. This skill is important for financial analysts and investment managers, as it ensures optimal asset allocation, risk management, and maximized returns.
- Stress TestingStress Testing is the process of evaluating a system's performance under extreme conditions. This skill is important for roles in finance and IT, ensuring resilience and risk management.
- Default PredictionDefault Prediction is the ability to forecast the likelihood of a borrower defaulting on a loan. this skill is important for risk analysts and financial managers to mitigate losses.
Key Learning Outcomes of Credit Risk Modeling Training Workshop for Employees
Edstellar’s Credit Risk Modeling training for employees will not only help your teams to acquire fundamental skills but also attain invaluable learning outcomes, enhancing their proficiency and enabling application of knowledge in a professional environment. By completing our Credit Risk Modeling workshop, teams will to master essential Credit Risk Modeling and also focus on introducing key concepts and principles related to Credit Risk Modeling at work.
Employees who complete Credit Risk Modeling training will be able to:
- Apply advanced statistical techniques to develop and refine credit scoring models, enabling precise risk assessment and decision-making in lending processes
- Analyze financial data to identify key predictors of credit risk using tools like logistic regression and decision trees, enhancing the accuracy of credit evaluations
- Develop comprehensive bankruptcy prediction models that integrate economic and behavioral data, helping institutions proactively manage and mitigate potential defaults
- Implement data preprocessing strategies to handle missing values and outliers, ensuring the reliability and validity of credit risk models
- Integrate machine learning techniques such as neural networks and survival analysis into credit risk modeling, providing a deeper insight into risk patterns and improving predictive power
Key Benefits of the Credit Risk Modeling Group Training
Attending our Credit Risk Modeling classes tailored for corporations offers numerous advantages. Through our Credit Risk Modeling group training classes, participants will gain confidence and comprehensive insights, enhance their skills, and gain a deeper understanding of Credit Risk Modeling.
- Learn to effectively apply various credit risk modeling techniques such as application, behavioral, and dynamic scoring to optimize lending decisions and manage financial risks
- Develop an understanding of bankruptcy prediction models, enabling you to identify early signs of financial distress and take preemptive actions to mitigate risks
- Explore expert models and credit ratings to gain insights into advanced risk assessment methods used by top rating agencies
- Learn how regulatory frameworks like Basel I, II, and III influence credit risk management and apply these standards to ensure compliance and enhance operational risk handling
- Equip teams with practical sampling and data preprocessing tools, crucial for building reliable and efficient predictive models in credit risk
Topics and Outline of Credit Risk Modeling Training
Our virtual and on-premise Credit Risk Modeling training curriculum is divided into multiple modules designed by industry experts. This Credit Risk Modeling training for organizations provides an interactive learning experience focused on the dynamic demands of the field, making it relevant and practical.
- Application scoring
- Definitions and key concepts
- Models and applications
- Behavioral scoring
- Overview and methodology
- Impact on credit decisions
- Dynamic scoring
- Fundamentals and development
- Practical applications in credit risk
- Credit bureaus
- Role in credit scoring
- Interaction with financial institutions
- Bankruptcy prediction models
- Techniques and indicators
- Expert models
- Design and implementation strategies
- Credit ratings and rating agencies
- Functions and influence on credit markets
- Regulation and accuracy issues
- Regulatory versus Economic capital
- Definitions and distinctions
- Implications for financial institutions
- Basel I, Basel II, and Basel III regulations
- Overview of each framework
- Evolution of banking regulations
- Standard approach versus IRB approaches for credit risk
- Comparative analysis
- Benefits and challenges of each approach
- PD versus LGD versus EAD
- Definitions and importance in credit risk management
- Interaction and impact on financial modeling
- Expected loss versus unexpected loss
- Conceptual understanding
- Calculation methods and relevance
- The Merton/Vasicek model
- Theoretical foundations
- Application in credit risk modeling
- Selecting the sample
- Criteria and strategies
- Impact on model accuracy
- Types of variables
- Classification and significance
- Handling different data types
- Missing values (imputation schemes)
- Common techniques for handling missing data
- Effects on model reliability
- Outlier detection and treatment
- Identification methods
- Correction strategies
- Exploratory data analysis
- Tools and techniques
- Role in model development
- Categorization (chi-squared analysis, odds plots, etc)
- Methods and applications
- Importance in variable selection
- Weight of evidence (WOE) coding and information value (IV)
- Calculation and interpretation
- Use in predictive modeling
- Segmentation
- Approaches and benefits
- Reject inference
- Concept and methodologies
- Importance in credit scoring models
- Basic concepts of classification
- Overview and key terms
- Importance in credit risk
- Classification techniques: Logistic regression, decision trees, linear programming, k-nearest neighbor, cumulative logistic regression
- Description and comparison of each technique
- Selection criteria based on data characteristics
- Input selection methods such as filters, forward/backward/stepwise regression, and p-values
- Techniques and their impact on model performance
- Setting the cutoff (strategy curve, marginal good-bad rates)
- Importance of cutoff setting in decision-making
- Techniques for optimal cutoff determination
- Measuring scorecard performance
- Key metrics and their interpretations
- Tools for performance evaluation
- Splitting up the data: Single sample, holdout sample, cross-validation
- Advantages and disadvantages of each method
- Performance metrics such as ROC curve, CAP curve, and KS statistic
- Overview and calculation methods
- Importance in model validation
- Defining ratings
- Process and criteria for rating assignments
- Impact on credit decisions
- Migration matrices
- Concept and construction
- Uses in credit risk management
- Rating philosophy (Point-in-Time versus Through-the-Cycle)
- Definitions and differences
- Strategic implications for financial institutions
- Mobility metrics
- Overview and applications
- Role in dynamic credit modeling
- PD calibration
- Techniques and importance
- Challenges in calibration processes
- Scorecard alignment and implementation
- Strategies for effective integration
- Challenges and solutions in practical implementation
- Modeling Loss Given Default (LGD)
- Introduction to concepts and methodologies
- Approaches to LGD estimation
- Defining LGD using the market approach and workout approach
- Comparison and practical applications
- Benefits and limitations of each method
- Choosing the workout period
- Factors influencing the selection
- Impact on LGD calculation
- Dealing with incomplete workouts
- Strategies for handling data gaps
- Effects on LGD accuracy
- Setting the discount factor
- Importance in present value calculations
- Methods for determining appropriate rates
- Calculating indirect costs
- Identifying and quantifying hidden expenses
- Inclusion in total loss calculations
- Drivers of LGD
- Key factors affecting loss severities
- Modeling LGD using segmentation (expert-based versus regression trees)
- Techniques and decision criteria for segmentation
- Comparative analysis of approaches
- Modeling LGD using linear regression
- Application in loss prediction
- Advantages and challenges
- Shaping the Beta distribution for LGD
- Statistical foundations and applications
- Fitting models to loss data
- Modeling LGD using two-stage models
- Design and implementation
- Benefits over traditional single-stage models
- Measuring the performance of LGD models
- Metrics and benchmarks
- Continuous improvement processes
- Defining LGD ratings
- Criteria for rating classifications
- Use in risk management frameworks
- Calibrating LGD
- Techniques and calibration processes
- Importance of accuracy and precision
- Default-weighted versus exposure-weighted versus time-weighted LGD
- Definitions
- Selection criteria based on portfolio characteristics
- Economic downturn LGD
- Concept and significance
- Predicting and preparing for recession impacts
- Modeling exposure at default (EAD)
- Overview of methods and importance
- Estimation challenges and solutions
- Defining CCF (credit conversion factors)
- Introduction to Credit Conversion Factors
- Importance in credit exposure estimation
- Cohort/fixed time horizon/momentum approach for CCF
- Methodologies and selection
- Application in different credit environments
- Risk drivers for CCF
- Identifying and quantifying influences on CCF
- Impact on overall credit risk management
- Modeling CCF using segmentation and regression approaches
- Application techniques and scenarios
- Advantages of each method
- CAP curves for LGD and CCF
- Constructing and interpreting CAP curves
- Use in performance evaluation
- Correlations between PD, LGD, and EAD
- Exploring interdependencies
- Impact on risk quantification and management
- Calculating expected loss (EL)
- Formulae and computational methods
- Strategic importance in financial planning
- Validating PD, LGD, and EAD models
- Standards and methodologies for validation
- Regulatory requirements and compliance
- Quantitative versus qualitative validation
- Differences and applications
- Balancing quantitative data with qualitative insights
- Backtesting for PD, LGD, and EAD
- Techniques and frequency
- Role in model governance
- Backtesting model stability (system stability index)
- Metrics for assessing model stability
- Strategies for maintaining consistency over time
- Backtesting model discrimination (ROC, CAP, overrides, etc.)
- Evaluating model discrimination capabilities
- Importance in risk differentiation
- Backtesting model calibration using the binomial, Vasicek, and chi-squared tests
- Calibration tests and their applications
- Importance in maintaining model accuracy
- Traffic light indicator approach
- System design and implementation
- Use in monitoring model performance
- Backtesting action plans
- Developing and implementing remedial actions
- Importance in continuous improvement cycles
- Through-the-cycle (TTC) versus point-in-time (PIT) validation
- Comparison of approaches
- Choosing the right method for the portfolio
- Benchmarking
- Internal versus external benchmarking
- Techniques and benefits of benchmark comparisons
- Kendall's tau and Kruskal's gamma for benchmarking
- Statistical tools for correlation analysis
- Application in risk model benchmarking
- Use testing
- Practical testing of model applications
- Data quality
- Standards and practices for ensuring high-quality data
- Impact on model reliability
- Documentation
- Requirements for comprehensive documentation
- Role in audit and regulatory compliance
- Corporate governance and management oversight
- Governance structures for risk modeling
- Oversight mechanisms and their importance
- Definition of LDP
- Understanding the characteristics of Low Default Portfolios
- Importance in risk assessment
- Sampling approaches (undersampling versus oversampling)
- Techniques and rationale for each approach
- Impact on model accuracy and reliability
- Likelihood approaches
- Statistical methods for dealing with low default data
- Application in credit risk models
- Calibration for LDPs
- Strategies for effective calibration
- Challenges and solutions in LDP contexts
- Overview of stress testing regulation
- Regulatory framework and compliance requirements
- Evolution and future trends in stress testing
- Sensitivity analysis
- Techniques and applications
- Importance in understanding model robustness
- Scenario analysis (historical versus hypothetical)
- Designing and implementing scenario analyses
- Comparison of historical data with hypothetical situations
- Pillar 1 versus Pillar 2 stress testing
- Distinctions and implications for financial institutions
- Strategic importance in risk management frameworks
- Macro-economic stress testing
- Integrating macro-economic factors into stress tests
- Background
- Introduction to neural networks in credit scoring
- Evolution and current trends
- The multilayer perceptron (MLP)
- Structure and function of MLPs
- Applications in credit risk modeling
- Transfer functions
- Overview of transfer functions in neural networks
- Role in signal processing and model accuracy
- Data preprocessing
- Necessary steps for preparing data for neural network models
- Impact on model performance
- Weight learning
- Techniques for optimizing weights in neural networks
- Overfitting
- Identification and mitigation strategies
- Long-term impacts on model utility
- Architecture selection
- Criteria for choosing the right neural network architecture
- Trade-offs involved in model complexity
- Opening the black box
- Techniques to increase transparency in neural network models
- Importance in regulatory and operational contexts
- Self Organizing Maps (SOMs)
- Introduction to SOMs and their unique properties
- Using SOMs in credit risk identification and management
- Survival analysis for credit scoring
- Fundamentals of survival analysis in the context of credit risk
- Key benefits and implementation challenges
- Censoring
- Understanding the concept of censoring in survival data
- Methods to handle censored data in analysis
- Time-varying covariates
- Role and handling of covariates that change over time
- Impact on survival analysis accuracy
- Survival distributions
- Commonly used survival distributions in credit scoring
- Selection and application of appropriate models
- Kaplan-Meier analysis
- Performing and interpreting Kaplan-Meier survival estimates
- Comparison with other survival analysis methods
- Parametric survival analysis
- Advantages of parametric methods over non-parametric
- Types of parametric models and their applications
- Proportional hazards regression
- Overview of the Cox proportional hazards model
- Applications in credit risk modeling
- Discrete survival analysis
- Distinct features and methodology
- Evaluating Survival Analysis Models
- Metrics and methods for assessing model performance
- Continuous improvement and validation strategies
- Competing risks
- Introduction to the concept of competing risks in survival analysis
- Methodological approaches and practical implications
- Mixture cure modeling
- Explanation of mixture cure models and their relevance
- Application in credit risk scenarios with partial recoveries
Who Can Take the Credit Risk Modeling Training Course
The Credit Risk Modeling training program can also be taken by professionals at various levels in the organization.
- Credit Analysts
- Risk Analysts
- Financial Analysts
- Investment Analysts
- Data Analysts
- Portfolio Specialists
- Finance Professionals
- Credit Risk Analysts
- Quantitative Analysts
- Credit Officers
- Data Scientists
- Managers
Prerequisites for Credit Risk Modeling Training
Employees with a basic understanding of financial principles and risk management can take the Credit Risk Modeling training course.
Corporate Group Training Delivery Modes
for Credit Risk Modeling Training
At Edstellar, we understand the importance of impactful and engaging training for employees. As a leading Credit Risk Modeling training provider, we ensure the training is more interactive by offering Face-to-Face onsite/in-house or virtual/online sessions for companies. This approach has proven to be effective, outcome-oriented, and produces a well-rounded training experience for your teams.
.webp)
Edstellar's Credit Risk Modeling virtual/online training sessions bring expert-led, high-quality training to your teams anywhere, ensuring consistency and seamless integration into their schedules.
.webp)
Edstellar's Credit Risk Modeling inhouse training delivers immersive and insightful learning experiences right in the comfort of your office.
.webp)
Edstellar's Credit Risk Modeling offsite group training offer a unique opportunity for teams to immerse themselves in focused and dynamic learning environments away from their usual workplace distractions.
Explore Our Customized Pricing Package
for
Credit Risk Modeling Corporate Training
Need the cost or quote for onsite, in-house, or virtual instructor-led corporate Credit Risk Modeling training? Get a customized proposal that fits your team's specific needs.
64 hours of group training (includes VILT/In-person On-site)
Tailored for SMBs
Tailor-Made Trainee Licenses with Our Exclusive Training Packages!
160 hours of group training (includes VILT/In-person On-site)
Ideal for growing SMBs
Tailor-Made Trainee Licenses with Our Exclusive Training Packages!
400 hours of group training (includes VILT/In-person On-site)
Designed for large corporations
Tailor-Made Trainee Licenses with Our Exclusive Training Packages!
Unlimited duration
Designed for large corporations
Edstellar: Your Go-to Credit Risk Modeling Training Company
Experienced Trainers
Our trainers bring years of industry expertise to ensure the training is practical and impactful.
Quality Training
With a strong track record of delivering training worldwide, Edstellar maintains its reputation for its quality and training engagement.
Industry-Relevant Curriculum
Our course is designed by experts and is tailored to meet the demands of the current industry.
Customizable Training
Our course can be customized to meet the unique needs and goals of your organization.
Comprehensive Support
We provide pre and post training support to your organization to ensure a complete learning experience.
Multilingual Training Capabilities
We offer training in multiple languages to cater to diverse and global teams.
What Our Clients Say
We pride ourselves on delivering exceptional training solutions. Here's what our clients have to say about their experiences with Edstellar.
"Edstellar's IT Service Management training has been transformative. Our IT teams have seen significant improvements through multiple courses delivered at our office by expert trainers. Excellent feedback has prompted us to extend the training to other teams."
"Edstellar's quality and process improvement training courses have been fantastic for our team of quality engineers, process engineers and production managers. It’s helped us improve quality and streamline manufacturing processes. Looking ahead, we’re excited about taking advanced courses in quality management, and project management, to keep improving in the upcoming months."
"Partnering with Edstellar for web development training was crucial for our project requirements. The training has equipped our developers with the necessary skills to excel in these technologies. We're excited about the improved productivity and quality in our projects and plan to continue with advanced courses."
"Partnering with Edstellar for onsite ITSM training courses was transformative. The training was taken by around 80 IT service managers, project managers, and operations managers, over 6 months. This has significantly improved our service delivery and standardized our processes. We’ve planned the future training sessions with the company."
"Partnering with Edstellar for onsite training has made a major impact on our team. Our team, including quality assurance, customer support, and finance professionals have greatly benefited. We've completed three training sessions, and Edstellar has proven to be a reliable training partner. We're excited for future sessions."
"Edstellar's online training on quality management was excellent for our quality engineers and plant managers. The scheduling and coordination of training sessions was smooth. The skills gained have been successfully implemented at our plant, enhancing our operations. We're looking forward to future training sessions."
"Edstellar's online AI and Robotics training was fantastic for our 15 engineers and technical specialists. The expert trainers and flexible scheduling across different time zones were perfect for our global team. We're thrilled with the results and look forward to future sessions."
"Edstellar's onsite process improvement training was fantastic for our team of 20 members, including managers from manufacturing, and supply chain management. The innovative approach, and comprehensive case studies with real-life examples were highly appreciated. We're excited about the skills gained and look forward to future training."
"Edstellar's professional development training courses were fantastic for our 50+ team members, including developers, project managers, and consultants. The multiple online sessions delivered over several months were well-coordinated, and the trainer's methodologies were highly effective. We're excited to continue our annual training with Edstellar."
"Edstellar's IT service management training for our 30 team members, including IT managers, support staff, and network engineers, was outstanding. The onsite sessions conducted over three months were well-organized, and it helped our team take the exams. We are happy about the training and look forward to future collaborations."
"Edstellar's office productivity training for our 40+ executives, including project managers and business analysts, was exceptional. The onsite sessions were well-organized, teaching effective tool use with practical approaches and relevant case studies. Everyone was delighted with the training, and we're eager for more future sessions."
"Edstellar's quality management training over 8 months for our 15+ engineers and quality control specialists was outstanding. The courses addressed our need for improved diagnostic solutions, and the online sessions were well-organized and effectively managed. We're thrilled with the results and look forward to more."
"Edstellar's digital marketing training for our small team of 10, including content writers, SEO analysts, and digital marketers, was exactly what we needed. The courses delivered over a few months addressed our SEO needs, and the online sessions were well-managed. We're very happy with the results and look forward to more."
"Edstellar's telecommunications training was perfect for our small team of 12 network engineers and system architects. The multiple online courses delivered over a few months addressed our needs for network optimization and cloud deployment. The training was well-managed, and the case studies were very insightful. We're thrilled with the outcome."
"Edstellar's professional development training was fantastic for our 50+ participants, including team leaders, analysts, and support staff. Over several months, multiple courses were well-managed and delivered as per the plan. The trainers effectively explained topics with insightful case studies and exercises. We're happy with the training and look forward to more."
Get Your Team Members Recognized with Edstellar’s Course Certificate
Upon successful completion of the Credit Risk Modeling training course offered by Edstellar, employees receive a course completion certificate, symbolizing their dedication to ongoing learning and professional development.
This certificate validates the employee's acquired skills and is a powerful motivator, inspiring them to enhance their expertise further and contribute effectively to organizational success.

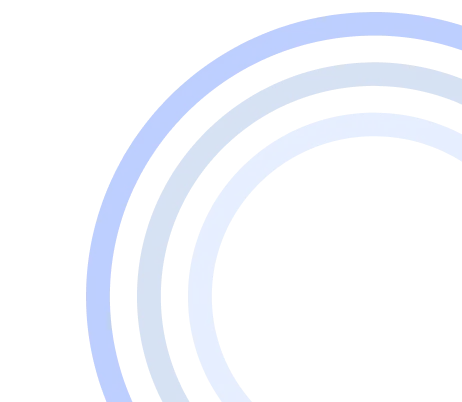
We have Expert Trainers to Meet Your Credit Risk Modeling Training Needs
The instructor-led training is conducted by certified trainers with extensive expertise in the field. Participants will benefit from the instructor's vast knowledge, gaining valuable insights and practical skills essential for success in Access practices.