
Ensemble Learning skills corporate training will enable teams to effectively apply their learnings at work.
- Data Scientists
- Machine Learning Engineers
- AI Specialists
- Data Analysts
- Research Analysts
- Software Developers
- Business Intelligence Analysts
- System Architects
- Data Engineers
- Data Architects
- IT Directors
- Project Managers
64 hours of group training (includes VILT/In-person On-site)
Tailored for SMBs
160 hours of group training (includes VILT/In-person On-site)
Ideal for growing SMBs
Tailor-Made Trainee Licenses with Our Exclusive Training Packages!
400 hours of group training (includes VILT/In-person On-site)
Designed for large corporations
Tailor-Made Trainee Licenses with Our Exclusive Training Packages!
Unlimited duration
Designed for large corporations
Experienced Trainers
Our trainers bring years of industry expertise to ensure the training is practical and impactful.
Quality Training
With a strong track record of delivering training worldwide, Edstellar maintains its reputation for its quality and training engagement.
Industry-Relevant Curriculum
Our course is designed by experts and is tailored to meet the demands of the current industry.
Customizable Training
Our course can be customized to meet the unique needs and goals of your organization.
Comprehensive Support
We provide pre and post training support to your organization to ensure a complete learning experience.
Multilingual Training Capabilities
We offer training in multiple languages to cater to diverse and global teams.
What Our Clients Say
We pride ourselves on delivering exceptional training solutions. Here's what our clients have to say about their experiences with Edstellar.
Get Your Team Members Recognized with Edstellar’s Course Certificate

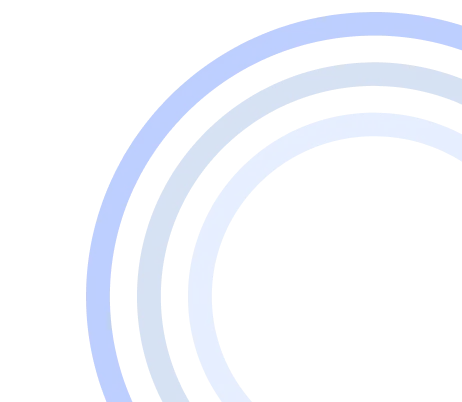