Most data analytics teams don’t fail because of bad tools they fail because they weren’t built with a clear strategy. It’s no surprise that 84% of CEOs express concern over the quality of information driving their decisions. The problem isn’t data access it’s what teams are (or aren’t) doing with it.
The pressure to become data-driven leads many companies to jump in too fast. They rush to hire data scientists or deploy dashboards. But months later, they realize something’s not working insights aren’t driving decisions, stakeholders aren’t aligned, and the analytics team is stuck reacting to scattered requests instead of solving meaningful business problems.
And this misalignment is costly. Even as global spending on data analytics is set to surpass $82 billion in 2025, few organizations consistently extract measurable value. Why? Because the issue isn’t lack of investment it’s the absence of a team structure built to turn data into action.
The irony is, we’re in the most advanced era of analytics to date. Platforms like Microsoft Fabric eliminate silos across data engineering, transformation, real-time analytics, and AI workflows. Copilot integrations are accelerating how quickly teams can generate insights. Yet, despite this progress, the advantage still depends on one thing: the team’s ability to connect technology to business outcomes.
As organizations scale, general data literacy is no longer enough. What’s needed is a purpose-built analytics team engineers, analysts, scientists, and strategists working in sync, with the clarity and structure to align efforts with business goals. It’s no surprise that 82% of companies plan to increase investment in analytics in 2025.
This guide breaks down seven strategic steps to help you build a high-impact data analytics team designed for scale, accountability, and measurable business impact.
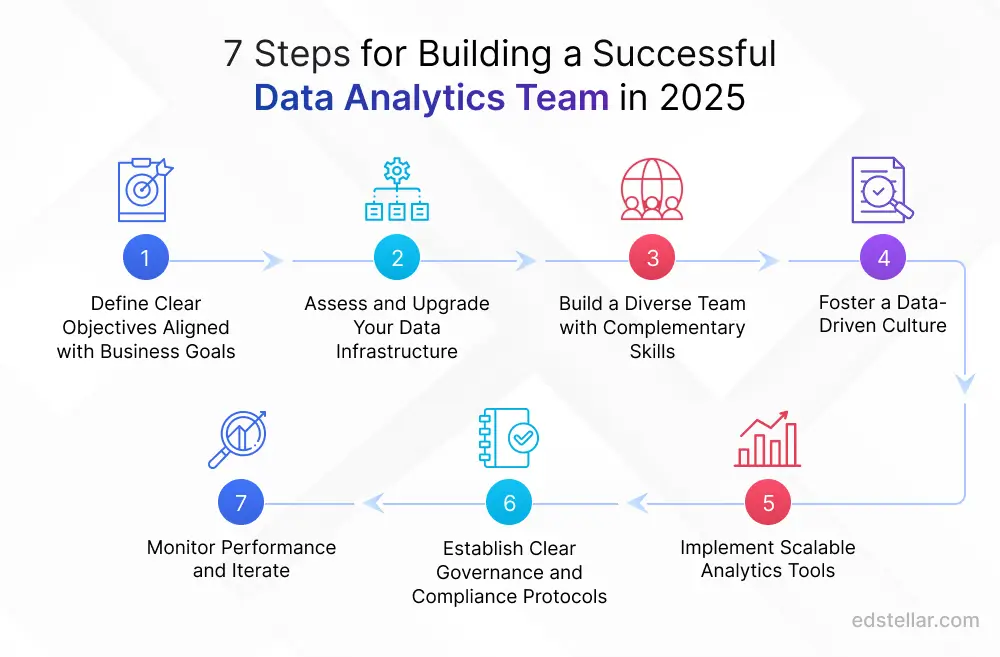
Step 1: Define Clear Objectives Aligned with Business Goals
Before building your data analytics team, it's essential to clearly define objectives that align with your organization's overall business strategy. The “why” behind your analytics initiative will directly influence the kind of talent you need, the tools you adopt, and how your team operates.
For instance, if your goal is to enhance customer experience, your team might focus on real-time analytics, customer segmentation, and behavioral tracking, skills typically found in analysts with a strong grasp of customer data platforms and personalization models. On the other hand, if your priority is driving innovation or optimizing operations, your team may need strengths in process automation, operational KPIs, or product lifecycle analytics.
Key Measures to Get Alignment Right:
Clarify the Primary Business Problem You’re Solving
- Define what business outcome you’re aiming for (e.g., improve churn rate, reduce operational costs, increase customer LTV).
- Ask: What decisions do we want to enable or improve with analytics?
Translate Strategic Goals into Measurable Analytics Use Cases
- Break down abstract business goals into analytics-driven questions or KPIs (e.g., "How can we improve order fulfillment time by 15%?”).
- Prioritize use cases based on impact and feasibility.
Engage Functional Leaders Early in the Planning Process
- Include stakeholders from marketing, finance, operations, sales, and product in early workshops or discovery calls.
- Capture both cross-functional pain points and expectations from analytics.
Determine Which Data Signals Matter Most to Each Team
- Identify critical data inputs each department relies on e.g., marketing may need customer attribution data, while ops may need supply chain visibility.
- This helps ensure your team isn’t collecting data just because it’s available but because it’s useful.
Assess Current Data Maturity and Readiness
- Evaluate existing data infrastructure, data literacy, and culture across teams.
- Helps avoid setting goals that are too advanced for current capabilities or too basic to deliver ROI.
Set Success Metrics Aligned with Business Language
- Define success not just in terms of reports generated or dashboards built, but in business terms (e.g., % increase in retention, $ savings from forecast accuracy).
- This creates shared accountability between analytics and business teams.
Step 2: Assess and Upgrade Your Data Infrastructure
A scalable and modern data infrastructure is essential for high-performing analytics teams. Begin by evaluating your existing data environment: Are your storage systems scalable? Can your data pipelines support real-time analytics? Are your integration tools robust enough for disparate data sources? Modern architectures like cloud-native platforms (e.g., Snowflake, Google BigQuery, Amazon Redshift) and data lakes support agility, reduce latency, and offer better storage economics.
98% of organizations globally are now using some form of cloud services ranging from SaaS tools to fully cloud-native infrastructures. Cloud Security Alliance
What This Phase Involves and What It Delivers
- Audit Your Current Data Environment
Outcome: Identifies system bottlenecks, legacy limitations, and readiness gaps that could slow down analytics performance. - Evaluate Scalability of Storage and Compute Systems
Outcome: Ensures your infrastructure can grow with data volume and team needs avoiding system slowdowns and rework later. - Assess Real-Time Data Pipeline Capabilities
Outcome: Determines if your team can support real-time use cases like live dashboards, anomaly detection, or instant decisioning. - Check Integration Flexibility Across Data Sources
Outcome: Confirms that your infrastructure can easily connect to CRMs, ERPs, marketing platforms, and external APIs breaking down data silos. - Adopt Cloud-Native Architectures (e.g., Snowflake, BigQuery, Redshift)
Outcome: Provides agility, cost-efficiency, and on-demand scalability for high-volume analytics workloads. - Implement or Optimize ETL/ELT Workflows (e.g., Fivetran, Apache NiFi, Azure Data Factory)
Outcome: Enables smooth, automated movement of data into your warehouse improving data freshness and reducing manual overhead. - Centralize Data Access and Governance
Outcome: Gives your team a single source of truth with the right access controls enhancing trust, security, and collaboration across departments.
Step 3: Build a Diverse Team with Complementary Skills
A successful analytics team requires a blend of technical, analytical, and business acumen. Include roles such as: Data engineers (pipeline and infrastructure), Data scientists (advanced modeling), Analysts (insights and reporting), Domain experts (industry knowledge), and Data architects (system design).
A high-performing analytics team isn’t just a collection of roles it’s a blend of perspectives, skill sets, and problem-solving approaches. When you bring together individuals with varied technical strengths, domain expertise, and ways of thinking, you minimize blind spots, challenge assumptions, and build analytics solutions that are more accurate, ethical, and aligned with business realities.
Pairing data scientists with domain experts helps ensure that models reflect the real-world context they’re meant to support. Involving analysts with strong communication skills ensures insights are generated and clearly understood and acted upon. This diversity of expertise fosters better decision-making and consistently drives more meaningful outcomes from your analytics initiatives.
Step 4: Foster a Data-Driven Culture
Building a team is not enough if the organization doesn’t embrace a data-first mindset. To truly unlock the value of analytics, the entire workforce, especially those on the front lines, must be empowered to make informed decisions based on data. Nearly 90% of organizations say their success depends on data-driven decisions made by frontline employees, according to businesswire.
Key Steps to Foster a Data-Driven Culture:
1. Promote Data Literacy Across the Organization
Launch foundational and role-specific training sessions to help employees understand, interpret, and question data.
Why it matters: Data-literate employees make faster, more confident decisions grounded in evidence not assumptions.
2. Offer Continuous Learning Through Workshops and Training Platforms
Use solutions like Edstellar to deliver instructor-led or self-paced learning based on departmental needs.
Why it matters: Ongoing learning ensures teams evolve alongside the data tools and strategies you adopt.
3. Run Department-Specific Analytics Sessions
Tailor training and use cases to show how analytics applies to each team’s goals (e.g., sales forecasting, campaign performance, supply chain efficiency).
Why it matters: Relevance boosts engagement and helps employees directly apply what they learn.
4. Embed Analytics Team Members Within Business Units
Assign data analysts or liaisons to specific functions like marketing, ops, or HR.
Why it matters: It builds trust, improves data interpretation, and ensures business context is always considered.
5. Appoint “Data Ambassadors” or Champions in Each Team
Identify motivated individuals who can promote data best practices, encourage usage, and act as the bridge between analytics and business users.
Why it matters: Peer advocacy is often more effective than top-down enforcement in changing habits.
6. Celebrate and Share Data-Driven Wins
Publicize successful projects or decisions that were backed by analytics.
Why it matters: Recognizing outcomes reinforces a culture where using data is seen as valuable and impactful.
Step 5: Implement Scalable Analytics Tools
The right tools drive performance. Select tools based on team maturity, business needs, and technical requirements. Visualization platforms like Tableau, Power BI, and Looker allow users to explore and present data intuitively. For more advanced use cases, Databricks and Apache Spark offer massive data processing and machine learning capabilities.
According to the IDC Worldwide Big Data and Analytics Forecast, spending on modern analytics tools is expected to reach $274.3 billion in 2025, highlighting the growing need for scalable solutions.
Choose tools that:
- Integrate with your data ecosystem
- Support real-time and batch processing
- Enable role-based access and collaboration
- Offer automation and AI-driven features
This ensures that your team can scale effortlessly as the business grows.
Step 6: Establish Clear Governance and Compliance Protocols
Without governance, analytics can lead to data chaos. Define clear policies around data access, ownership, quality, and lifecycle management. Data governance frameworks such as DAMA DMBOK or COBIT can provide a structured approach.
In 2023, IBM reported that poor data quality costs U.S. businesses over $3.1 trillion annually. This shows how critical structured governance is for maintaining data integrity. Also, stay up-to-date with compliance laws like: GDPR (EU), CCPA/CPRA (California), Australian Privacy Act (for AU businesses)
Leverage tools like Collibra, Alation, or Apache Atlas for data lineage, cataloging, and governance tracking. This protects your organization and instills confidence in your analytics outputs.
Step 7: Monitor Performance and Iterate
Analytics is not a “set and forget” initiative. Continuously evaluate your team’s performance using KPIs like:
- Speed to insight
- Forecast accuracy
- Impact on revenue or cost savings
- Adoption of analytics by departments
Feedback loops are essential. Schedule quarterly reviews, conduct team retrospectives, and stay in sync with evolving business needs. Use dashboards and tracking tools (e.g., Jira, Asana, or custom KPI portals) to manage goals and bottlenecks. Foster a culture of experimentation and improvement to keep your team agile and high-performing.
Case Study: Focus Brands Builds a High-Impact Data Analytics Team with Auxis
Background: Focus Brands is a prominent developer of global, multi-channel food service brands, overseeing over 6,400 restaurants, cafes, ice cream shops, and bakeries across the U.S., Puerto Rico, and more than 55 countries.
Challenge: With a vast international footprint, Focus Brands faced challenges in consolidating real-time data on sales, foot traffic, and brand quality across its 1,650+ international locations. The lack of integrated data hindered leadership's ability to make informed decisions regarding product mix, sales strategies, and business development opportunities.
Approach: To address these challenges, Focus Brands partnered with Auxis to build an advanced analytics team led by an in-house subject matter expert with a Ph.D. in data science. Key steps included:
- Identifying Key Data Dimensions: The team focused on four impactful data dimensions: sales, brand quality, product mix, and business development.
- Data Gap Analysis: Auxis conducted a thorough analysis to determine which business questions could be answered with existing data and identified necessary changes to improve data accuracy and timeliness.
- Implementation of Microsoft Power BI: A single Power BI app was developed to provide leadership with detailed and customizable visualizations, offering both high-level overviews and drill-down capabilities by market, country, region, or individual stores.
- Data Consolidation: Auxis consolidated data from various sources into a centralized data warehouse, ensuring data flows from a single location and is summarized appropriately.
Results: The advanced analytics program delivered real-time, accurate data that enhanced brand quality and sales. Notably, the program paid for itself within three months by boosting brand perception, sales performance, and optimizing product mix and promotions.
Conclusion
Building a high-performing data analytics team requires more than technical know-how; it demands strategy, structure, and continuous upskilling. Each step is critical to long-term success, from defining clear business-aligned objectives to implementing scalable tools and fostering a data-driven culture. As data environments grow more complex, investing in team training becomes non-negotiable.
Equip your teams with future-ready skills through Edstellar's Corporate Training Courses, designed specifically for data analytics groups. Empower your organization today with expert-led learning that accelerates data maturity and drives innovation.
Explore High-impact instructor-led training for your teams.
#On-site #Virtual #GroupTraining #Customized
Edstellar Training Catalog
Explore 2000+ industry ready instructor-led training programs.

Coaching that Unlocks Potential
Create dynamic leaders and cohesive teams. Learn more now!

Want to evaluate your team’s skill gaps?
Do a quick Skill gap analysis with Edstellar’s Free Skill Matrix tool

Transform Your L&D Strategy Today
Unlock premium resources, tools, and frameworks designed for HR and learning professionals. Our L&D Hub gives you everything needed to elevate your organization's training approach.
Access L&D Hub Resources